Today you are going to learn in betterthistechs article about The Generative Pre-trained Transformer (GPT) or generative artificial intelligence which is one of the most interesting and innovative new developments in the field of artificial intelligence. The GPT design has changed the field of Natural Language Processing (NLP) since it was first introduced in 2017. It has made way for a new era of intelligent language models. This article goes into great detail about how GPT works, how it has changed over time, what it can be used for, and how it has had a huge effect on many different businesses. So continue to read our betterthistechs article. let’s start
GPT Model | Release Year | Number of Parameters | Text Data Processed (Approx.) | Notable Features |
---|---|---|---|---|
GPT-1 | 2017 | 117 million | Huge Assumptions | Introduction of Transformer architecture |
GPT-2 | 2019 | 1.5 billion | Great Number of Implementations | Enhanced model size and performance |
GPT-3 | 2020 | 175 billion | Larger words Generated | Significantly larger model with improved language generation |
GPT-4 | 2022 | 1 trillion | Approx. Few billion words | Largest model to date, unparalleled language understanding |
Transformer’s Structure
The transformer, called the “king” of NLP systems, is at the heart of GPT. Transformers are different from their predecessors because they use self-attention mechanisms to process raw data at the same time. This lets them understand complex dependencies in text data. Because of this big step forward, Transformers is now very good at many NLP jobs, such as translating languages and making up text. This is the keen research of betterthistechs article.
Changes in GPT:Betterthistechs article
The first version of GPT came out in 2017, but it wasn’t until later versions like GPT-2 and GPT-3 that it really took off. The model got bigger and bigger with each run, and by the end, GPT-4 had an amazing one trillion parameters. This growth in size has greatly improved GPT’s success on many tasks, turning it into a strong AI contender.
Planning Ahead and Making Small Changes
According to our betterthistechs article A big part of GPT’s success is that it pre-trains models on huge amounts of text data, which gives them a deep knowledge of language. This model has already been trained and can be used as a starting point. It can then be tweaked to do specific jobs, like medical diagnosis or translating languages. Fine-tuning GPT lets it work well in a wide range of areas and really shine in specific tasks, showing how flexible and useful it is.
Getting Self-Supervised Learning Going
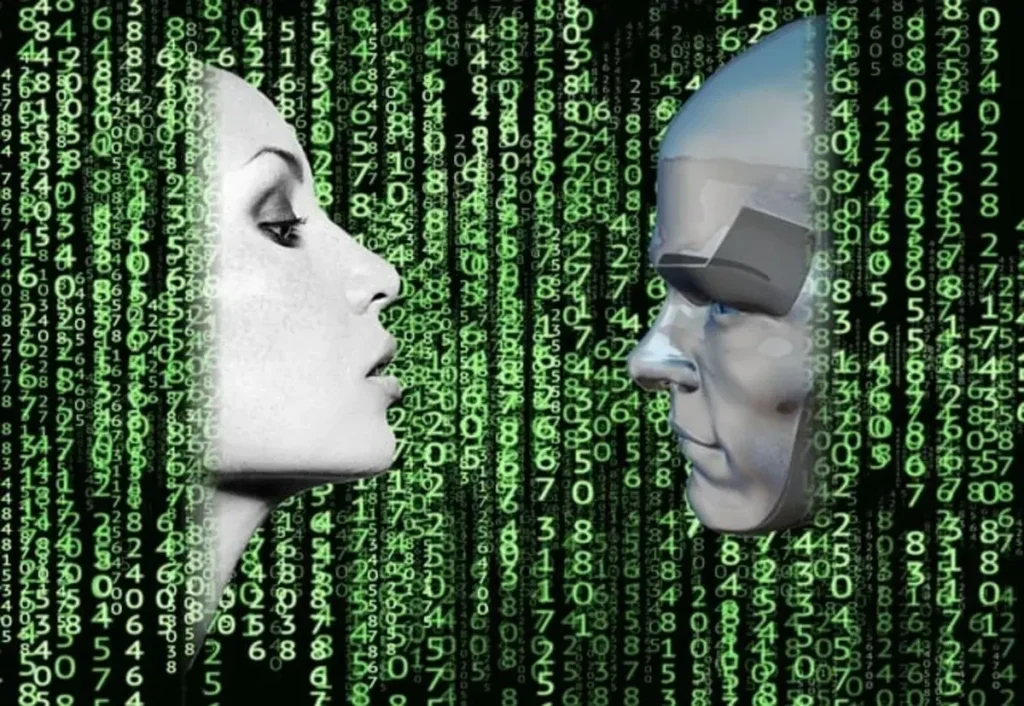
One of the most interesting things about GPT is that it uses self-supervised learning. GPT learns to write text that makes sense and is important to the situation by making predictions and trying again and again. This is similar to how humans understand. With this self-supervised method, GPT can keep improving its language creation skills, which is pushing the limits of what is possible in NLP.betterthistechs article wants to congratulate GPT team on their success related to this achievement of understanding from person to person.
Scaling Up: The Quest for Larger Models
In recent years, there has been a relentless pursuit of larger and more powerful language models, with GPT leading the charge(found by the research of our betterthistechs article). The exponential increase in model size, coupled with the sheer volume of text data processed during training, has propelled GPT to new heights of performance. However, this quest for scale raises questions about energy consumption and environmental impact, highlighting the need for responsible AI development.
Utilizing the Strength of Fine-tuning for Custom Use
Even though GPT’s pre-trained model can do a lot, its full potential can only be reached by fine-tuning it for specific tasks. Developers can make GPT work well in fields from healthcare to business by changing the model’s weights and parameters. This process of fine-tuning lets GPT solve problems in the real world with great accuracy and precision, which leads to new ideas in many fields.we will share more information in next betterthistechs article.
Dealing with Alignment Problems and Ethical Considerations
Our betterthistechs article will fully support It as it is important to deal with ethics issues and alignment problems as GPT continues to change. To make sure that AI systems follow human beliefs and intentions, they need to be used responsibly. The HHH framework, which stands for “Helpful, Honest, and Harmless,” is a way to reduce the risks of using AI and build trust between people and computers.
The Future of GPT: Chances and Difficulties Ahead
According to the research by our betterthistechs article GPT has a bright future ahead of it, but it also has a lot of problems to solve. As AI technology improves, it will be important to find a mix between new ideas and doing the right thing. Oversight and regulation will have a big impact on the direction of AI growth, making sure that its benefits are realized while minimizing its risks.
In conclusion
So betterthistechs article has fully explained that Generative In the area of Natural Language Processing, pre-trained Transformers are a turning point. From the beginning to now, GPT models have shown that they can understand and write text that sounds like it was written by a person. When we move on to the next stage of AI development, using GPT’s power in a smart way will be important for getting the most out of it and creating a world where people and machines can live together peacefully.
FAQs
- What is the significance of fine-tuning in AI models like GPT?
- Fine-tuning plays a crucial role in adapting pre-trained models like GPT for specific tasks. It involves initializing the model with pre-existing knowledge and then refining its parameters for the targeted task, such as medical diagnosis or language translation.
- How does the scale of AI models impact their performance?
- The size of AI models, measured by the number of parameters, has a significant impact on their performance. Larger models, such as GPT-4 with one trillion parameters, have shown a remarkable improvement in tasks compared to smaller versions like GPT-1 or GPT-2.
- What is the environmental impact of training and deploying AI models like GPT?
- Training and deploying large-scale AI models consume substantial computational resources, leading to significant energy consumption and carbon emissions. This environmental impact raises concerns about sustainability and calls for more efficient AI model architectures and training methodologies.
- How do AI models like GPT handle user instructions and tasks?
- AI models like GPT can be fine-tuned to follow user instructions and perform specific tasks by leveraging self-supervised learning and human-provided preferences. Through continuous refinement and feedback, these models aim to become more helpful, accurate, and aligned with user intents.
- What are the potential risks associated with the widespread adoption of AI models?
- While AI models like GPT offer numerous benefits, including automation and efficiency gains, they also pose risks such as misinformation propagation, job displacement, and unintended biases. Addressing these risks requires robust governance frameworks, ethical guidelines, and ongoing research efforts.
- How can society mitigate the risks posed by AI technologies?
- Mitigating the risks associated with AI technologies involves collaborative efforts from policymakers, researchers, industry stakeholders, and civil society. Key measures include implementing transparent and accountable AI governance mechanisms, fostering interdisciplinary research on AI ethics and safety, and promoting public awareness and digital literacy initiatives.
- What are the future prospects of AI technologies like GPT?
- The future of AI technologies like GPT holds both promise and challenges. While advancements in AI have the potential to revolutionize various sectors, including healthcare, education, and entertainment, they also raise complex ethical, legal, and societal implications that necessitate careful consideration and proactive mitigation strategies.